Blog
May 20, 2025
8 min read
What is Sales Performance Management (SPM)?
Blog
May 08, 2025
6 min read
How Managing Sales Territories Can Reduce Your Carbon Footprint
Blog
May 08, 2025
6 min read
The Psychology of Quota Planning for Quota Management: Balancing Goals and Achievability
Blog
May 07, 2025
7 min read
10 Things You Need in a Sales Performance Management (SPM) Platform
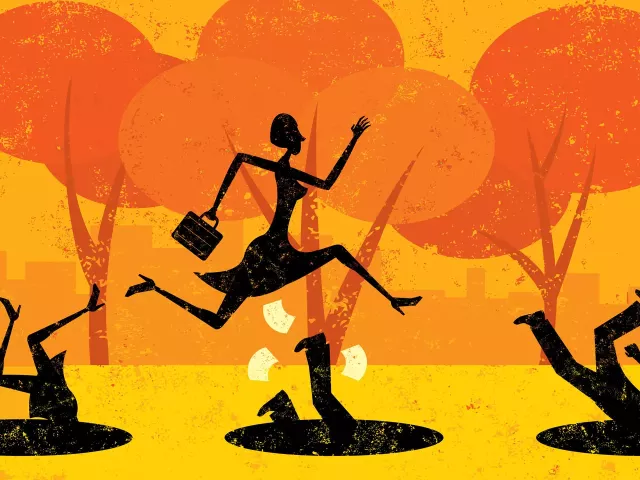
Blog
May 07, 2025
6 min read
Hidden Pitfalls of Territory Management (And How to Avoid Them)
Blog
Mar 28, 2025
3 min read
The Importance of Data in Agile Sales Planning: A Sales Ops Leader's Guide
Blog
Mar 25, 2025
3 min read
Xactly: Recognized as a Leader in IDC MarketScape and ISG Software Research Reports
Blog
Mar 10, 2025
2 min read
Driving Success in the Medical Device Industry: How Xactly Incent Transforms Sales Performance
Blog
Mar 07, 2025
2 min read