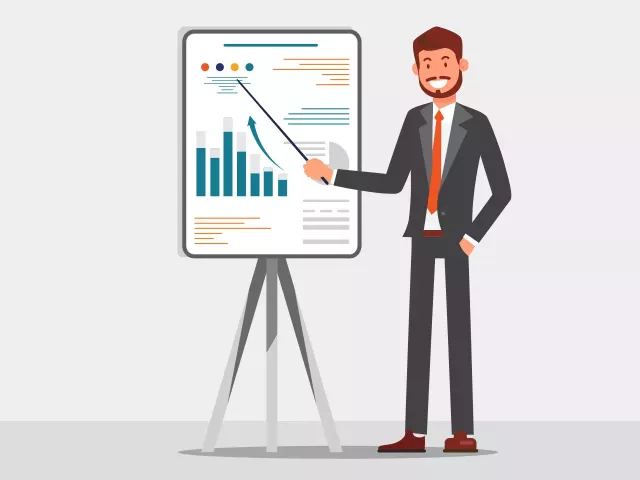
I have a theory – almost all forecasts are correct.
But there's a catch.
If you look at a high enough level (let’s say total company sales and not an individual territory) over a long enough period (a year or two rather than next month) and with a wide enough confidence interval (80% confidence rather than 95%) then you stand a pretty good chance of getting a forecast somewhere in the ballpark.
But does anyone find this useful at all? With a ton of small sales, the usefulness of an accurate forecast for an individual sale is low, and the above criteria may be good enough. But with a few large sales (i.e., enterprise) no one in their right mind would find this criteria useful. We want to know if we should include a big deal in the forecast and how much we expect from a few big clients. This is why almost all forecast tools available today fall short… and why the next wave of tools will change everything.
The Dark Ages
Let’s take a sample $1M deal in the pipeline. If we estimate that it has a 50% chance of closing, conventional forecasts will take the probability and multiply it by the deal value to arrive at a forecast. In this example, the forecast would be $500K. Conversely, most of the current forecasting tools will focus on refining the probability of the sale (i.e., the probability of the sale is 51% rather than 50%). If we look at all the deals across the company, this methodology can be used to arrive at an aggregate forecast. But that doesn’t help us if we want to look at this individual deal or territory. The simple truth is that there is no situation where the actual deal value would be $500K – it would either be $0 or $1M. Forecasting tools are not built to account for this – they are built to look across the entire organization and arrive at a number (the ballpark situation above).
How do you get an accurate forecast for a territory or a deal? Certain indicators can help refine the probability of a specific deal – historical rep ability to close, deal velocity, customer vertical, time of year – and if this data is available it can certainly help. Many forecast engines will adjust the probability of a deal up or down based on key indicators, but this is once again adjusting the probability up or down (i.e., the probability of the sale is 51% rather than 50%). Another way is to gather rep sentiment in the CRM, which can be surprisingly more accurate than using indicators to refine.
However, the most common methodology is to use deal stages to automatically assign a close probability. For example, deal stage 1 is assigned a 10% probability, stage 2 is assigned a 25% probability, and so on. The more sophisticated forecast models will wait until a deal hits a certain stage before including it in the forecast… by again multiplying the deal value by a certain probability. This methodology has the benefit of reducing the number of deals in the forecast to a smaller number of high-potential deals. However, this type of forecast is only good for a limited time frame, usually up to 90 days rather than for a full year.
The Better Method of Forecasting Enterprise Deals
A better forecast engine will only return a value of $0 or $1M in our example above. Therefore, the engine must be able to assign a deal value in the forecast of one or the other. Based on a deal score or probability the forecast engine can select to use either the full value or zero.
This is where AI can enhance a forecasting model as an even better forecast engine will leverage even more indicators to enhance the probability, and it will do this by analyzing the specific deals to find the highest correlated combination of indicators out of hundreds of options. It may even make suggestions on indicators that are not being used. In this way, the forecast engine will make an individualized forecast for each deal in the pipeline. For example, a deal enters the CRM and the forecast engine analyzes all the characteristics of the deal. It finds that the combination of predictors that yields the highest correlation are the business vertical, number of employees, number of online followers at the customer, recent purchases of supplies in the zip code, and time of year. Based on these predictors, the forecast engine predicts that there is a 60% probability that this deal will close. It returns a forecast value of 100%.
Since the AI can identify the indicators that yield the highest probability of closing it can be an invaluable tool for upstream marketing. The AI can identify the combinations of factors that marketing should target to generate the highest potential leads. This improves the lead-scoring process.
As the AI can identify the combination of indicators that should close it can be used to identify the sales representatives that continually fail to close those deals. In this way, the AI can be used to identify the representatives that fail to close the deals that should be won. That age-old question of ‘Is it the territory/lead flow or is it the rep’ gets answered (much to the chagrin of the Sales team).
Consider the Long Term
What if the company needs to set a full-year forecast? Surely, there are no leads in the pipeline for months 9 through 12 (or even 6 through 12). Companies currently need to rely on old-fashioned regression models or moving averages, or last year plus some growth. This is a bit ridiculous, as we are using AI to assign a deal probability to a lead in the pipeline, but if we want to take a longer look we are most likely using the equivalent of a chicken playing checkers.
But AI can assist here as well. While many different forecast models can be used (statistical and other), there are surprisingly few standard models with a myriad of variants. For example, a regression model forecast gives rise to exponential smoothing, ARIMA, vector autoregression, and decomposition models. Or a moving average model can give rise to seasonal adjustments, bootstrapping/Monte Carlo models. Each of these models relies on historical performance and AI can assess which model fits best based on this performance.;
For example, a company that sells cell phones sees steady growth for some time following each new release. This growth will start to fall and will only grow again following a new release. The AI would look at this type of historical performance and be able to identify that a Bass Diffusion model is the most accurate fit based on the standard models that it can use. It could then calculate the speed of customer adoption coefficient for the forecast and create a much more accurate forecast than the chicken playing checkers method. The AI can identify the best forecast model and apply it.
AI Will Continue to Improve Forecasting
The ability of AI to identify the best fit will continue to create better forecasts for leads in the pipeline as well as longer-term horizons. There is simply too much information being captured about prospects to create forecasts without AI. AI will feed on the information from the CRM and create links both upstream (marketing) and downstream (performance management). Companies that invest in technology early will have a distinct advantage in capturing high-potential clients and finding efficiencies in their organizations.
The content in this blog originally appeared on LinkedIn.